
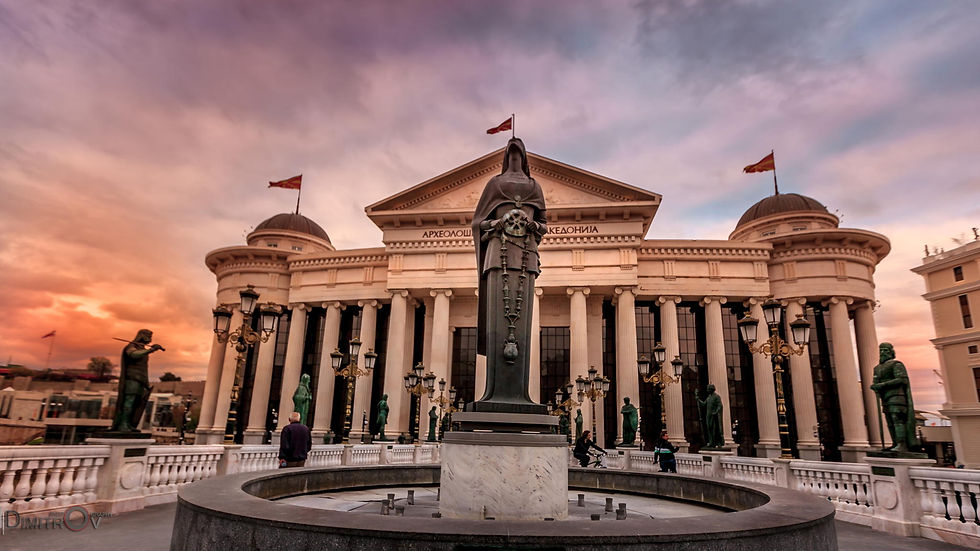
IoT
Large Scale
Learning from Data Streams
ECML-PKDD 2017
18 September 2017

IoT Stream for Data Driven Predictive Maintenance
​
Workshop+Tutorial
ECML-PKDD 2019
16 September 2019
The Workshop
Workshop + Tutorial
18 September 2017
09:00 am
The volume of data is rapidly increasing due to the development of the technology of information and communication. This data comes mostly in the form of streams. Learning from this ever-growing amount of data requires flexible learning models that self-adapt over time. In addition, these models must take into account many constraints: (pseudo) real-time processing, high-velocity, and dynamic multi-form change such as concept drift and novelty. This workshop welcomes novel research about learning from data streams in evolving environments. It will provide the researchers and participants with a forum for exchanging ideas, presenting recent advances and discussing challenges related to data streams processing. It solicits original work, already completed or in progress. Position papers are also considered. This workshop is combined with a tutorial treating the same topic and will be presented in the same day.
The Workshop
ECML/PKDD 2019 Workshop on
IoT Stream for Data Driven Predictive Maintenance
Workshop + Tutorial
16 September 2019
09:00 am
Maintenance is a critical issue in the industrial context for the prevention of high costs or injures.
The emerging technologies of Industry 4.0 empowered data production and exchange which
lead to new concepts and methodologies exploitation for maintenance. Intensive research effort
in data driven Predictive Maintenance (PdM) has been producing encouraged outcomes.
Therefore, the main objective of this workshop is to raise awareness of research trends and
promote interdisciplinary discussion in this field.
​
Motivation and focus
Maintenance is a crucial topic for industrial machines, medical equipment, energy systems,
passengers transport vehicles and home appliances among others. Cost reduction, machine
reliability, operation, safety and time reduction have been the main concerns of companies and
organizations. Meanwhile, Industry 4.0 brought new opportunities of meaningful data collection
and storage. Promising data-driven methodologies shrive for predictive maintenance becoming
a strong alternative.
This workshop will be centered on questions such as when to perform a maintenance action?
How to estimate components current and future status? How accurate are the existing
methods? Which data should be used? What decisions tools should be developed for
prognostic. How can Data Mining and Machine Learning (Artificial Intelligence in general)
contribute to answer these questions?
Therefore, this event is an opportunity to bridge researchers and engineers to discuss the
emerging topics and the key trends.
Aim and scope
This workshop solicits contributions including but not limited to the following topics:
-
Fault Detection and Diagnosis (FDD)
-
Fault Isolation and Identification
-
Estimation of Remaining Useful Life of Components, Machines, ….
-
Forecasting of Product and Process Quality
-
Early Failure and Anomaly Detection and Analysis
-
Automatic Process Optimization
-
Self-healing and Self-correction
-
Incremental, evolving (data-driven and hybrid) models for FDD and anomaly detection
-
Self-adaptive time-series based models for prognostics and forecasting
-
Adaptive signal processing techniques for FDD and forecasting
-
Concept Drift issues in dynamic predictive maintenance systems
-
Active learning and Design of Experiment (DoE) aspects in dynamic predictive maintenance
-
Systems Fault tolerant control
-
Decision Support Systems for Predictive Maintenance
-
Data visualization for Prescriptive Maintenance
-
Real world applications such as:
-
Manufacturing systems
-
Production Processes and Factories of the Future (FoF)
-
Wind turbines (offshore/onshore/floating)
-
Smart management of energy demand/response
-
Energy and power systems and networks
-
Transport systems
-
Power generation and distribution systems
-
Intrusion detection and cyber security
-
Internet of Things,
-
Next Generation Airspace Applications, etc.
-
Big Data challenges in energy transition and digital transition
-
Solar plant monitoring and management
-
Active demand response
-
Distributed renewable energy management and integration into smart grids
-
Smart cities
Submission and Review process
Regular and short papers presenting work completed or in progress are invited. Regular papers should not exceed 12 pages, while short papers are maximum 6 pages. Papers must be written in English and are to be submitted in PDF format online via the Easychair submission interface:
​
https://easychair.org/conferences/?conf=iotstream2019
Each submission will be evaluated on the basis of relevance, significance of contribution, quality of presentation and technical quality by at least two members of the program committee. All accepted papers will be included in the workshop proceedings and will be publically available on the conference web site. At least one author of each accepted paper is required to attend the workshop to present.
Important dates
Paper submission deadline: July 1st, 2019
Paper acceptance notification: July 19th, 2019
Paper camera-ready deadline: Monday, July 26th, 2019
Program Committee members (to be confirmed)
​
-
Carlos Ferreira, LIAAD INESC Porto LA, ISEP, Portugal
-
Edwin Lughofer, Johannes Kepler University of Linz, Austria
-
Sylvie Charbonnier, Université Joseph Fourier-Grenoble, France
-
Bruno Sielly Jales Costa, IFRN, Natal, Brazil
-
Fernando Gomide, University of Campinas, Brazil
-
José A. Iglesias, Universidad Carlos III de Madrid, Spain
-
Anthony Fleury, Mines-Douai, Institut Mines-Télécom, France
-
Teng Teck Hou, Nanyang Technological University, Singapore
-
Plamen Angelov, Lancaster University, UK
-
Igor Skrjanc, University of Ljubljana, Slovenia
-
Indre Zliobaite, University of Helsinki, Finland
-
Elaine Faria, Univ. Uberlandia, Brazil
-
Mykola Pechenizkiy, TU Eindonvhen, Netherlands
-
Raquel Sebastião, Univ. Aveiro, Portugal
Workshop Organizers
Rita Ribeiro
Laboratory of Artificial Intelligence and Decision Support, University of Porto, Porto, Portugalrpribeiro@dcc.fc.up.pt
Albert Bifet
Telecom-ParisTech; Paris, France
albert.bifet@telecom-paristech.fr
João Gama
Laboratory of Artificial Intelligence and Decision Support, University of Porto, Porto, Portugal
jgama@fep.up.pt
​
Anders Holst
RISE SICS
​
Sepideh Pashami
Halmstad University
​
​
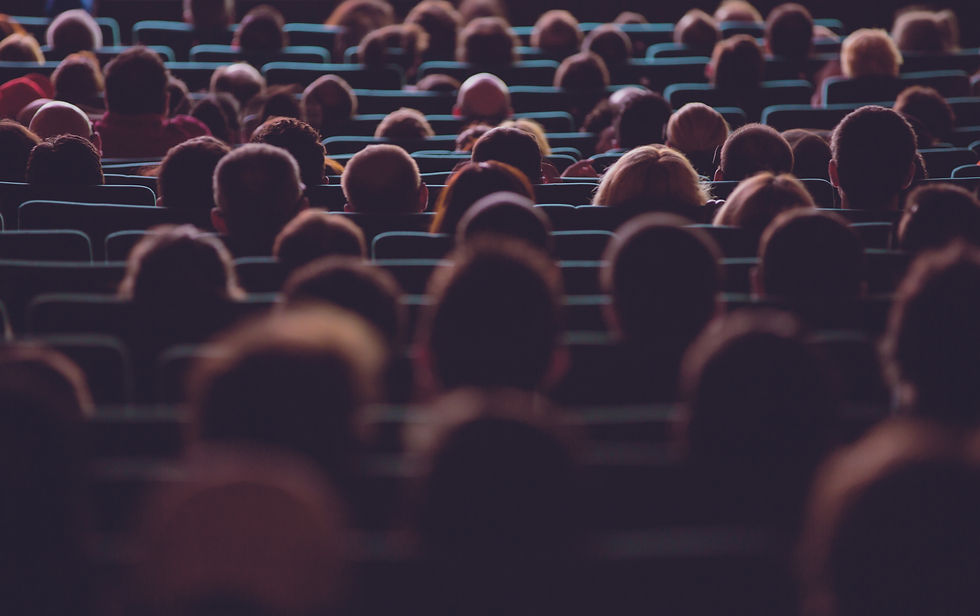
The Tutorial

Tutorial: IoT Data Stream Mining in Practice
The challenge of deriving insights from the Internet of Things (IoT) has
been recognized as one of the most exciting and key opportunities for both academia
and industry. The advent of IoT applications is here: industry 4.0, connected indus-
try, industry automation, smart cities, smart grids, energy efficiency, etc. All this IoT
applications require advanced analysis of big data streams from sensors and small
devices, while addressing security and privacy concerns. This tutorial is a gentle
introduction to mining IoT big data streams. The first part introduces data stream
learners for several learning tasks including distributed algorithms. The second and third part
present some applications for predictive maintenance, prediction for renewable ener-
gies, and social network analysis for telecommunications data streams. The last part presents how to use Apache Spark Streaming for applying scalable machine learning on Big Data streams.
​
Content:
​
1.IoT Fundamentals and IoT Stream Mining Algorithms
– Predictive Learning
– Descriptive Learning
– Frequent Pattern mining
2. Case Study: Predictive Maintenance
– Problem Definition
– Change, Anomaly and Novelty Detection
– Failure Prediction and Detection
3. Case Study: Social Network Analysis
– Challenges in mining networked data,
– Online sampling
– Evolving centralities and communities
– Tracking the dynamics of evolving communities
4. Big Data Stream Mining using Spark Streaming
– Fundamental concepts
– Examples
– API
​
Presenters:
​
-
Joao Gama
-
Rita Ribeiro
-
Moamar Sayed-Mouchaweh
-
Heitor Murilo Gomes
-
Latifur Khan
-
Albert Bifet
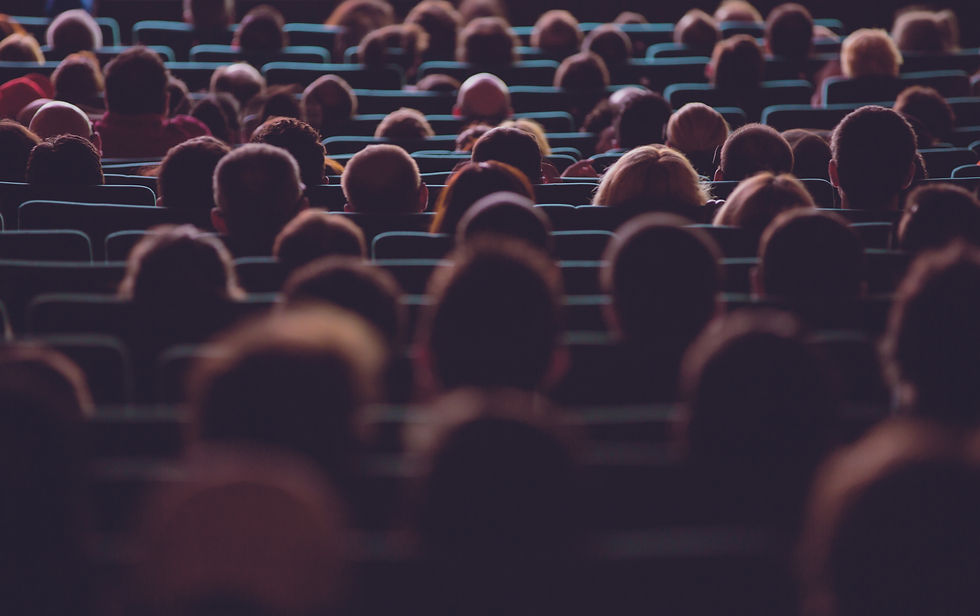
The Tutorial

Tutorial: Predictive maintenance for industrial complex systems: A case-study on predictive
maintenance of solar power plants within the context of energy transition
​
Slides: Part I, Part II and Part III
Predictive maintenance is a central cornerstone in today’s industrial applications and systems
ranging from on-line manufacturing rails and production lines through (cyber) security problems
to energy fabrication and exploitation facilities. This is because it addresses and develops not
only strategies for the early detection and prediction of machine failures, degraded performance,
undesired situations and occurrences or downtrends in product quality, but also for taking
appropriate actions upon the recognition and prediction of such occasions. Such actions are
indispensable for reducing waste, production costs, customer complaints and thus, in the long
run, also for increasing the income of companies, for guaranteeing higher quality of production
items, software and user frontends and finally for reducing the pollution of the environment. In
the extreme case of severe system failures, any risks of operators working with the system can
be avoided by predictive maintenance. High-qualitative methodologies and functioning
procedures for fully- or at least semi-automated tasks are required to assure efficiency and
economy of predictive maintenance systems. Self-adaptivity of the methods and models is
necessary to handle significant dynamics and non-stationary environments adequately. Recent
developments of proper methodologies to tackle these issues and their application in real-world
predictive maintenance installations will be the content of this tutorial concluded by a discussion
about future challenges and open issues. This subject has many applications with increasing
size such as production systems, energy production, energy distribution, energy management,
renewable energy production (utilities/distributed, offshore/onshore wind farms, solar centers),
cyber security, internet of things etc. A case-study related to the predictive maintenance of
renewable energy resources will be used through the tutorial in order to illustrate the different
notions, concepts, and presented methods.
​
Content:
​
1.Introduction
a.Definition and general principal
b.Challenges and motivation
c.Overview of real-world applications requiring predictive maintenance
2.Principal steps to perform the predictive maintenance
a.Data acquisition and pretreatment
b.Health indicator construction
c.Early fault detection and diagnosis
d.Degradation detection and mode determination
e.Fault prognosis and remaining useful life prediction
f.Predictive maintenance requirement, strategy and asset management
3.General principal and classification of approaches of predictive maintenance in industrial
complex systems
a.Physical model-based approaches
b.Data-driven based approaches
c.Artificial Intelligence approaches
d.Hybrid approaches
4.Metrics for predictive maintenance
5.Case study: predictive maintenance of solar farms
a.Introduction, motivation and challenges
b.Available data
c.Data preprocessing and preparation
d.Data labeling
e.Data analysis
f.Model design, validation and update
g.Experimentation and evaluation
6. Conclusion and discussion
​
Presenters:
​
-
Moamar Sayed-Mouchaweh
-
Ricardo Sousa
-
Slawomir Nowaczyk
Program
​
Room 1.010
09h-10:30 Tutorial: part I II p
​
10h30-11h Cofee Break
​
11h-12h40 Tutorial: part II
​
12h40-14h Snack Break
​
14h-14.45 Invited Talk - Myra Spiliopoulou: IoT and mHealth: Beyond Sensors
14h45-15h00 Pawel Zyblewski, Robert Sabourin and Michal Wozniak. Data preprocessing and dynamic ensemble selection for imbalanced data stream classification
15h00-15h20 Coffee Break
15h20-15h35 Mathias Van Herreweghe, Mathias Verbeke, Wannes Meert and Tom Jacobs. A Machine Learning-
based Approach for Predicting Tool Wear in Industrial Milling Processes
15h35-15h50 Athanasios Naskos, Georgia Kougka, Theodoros Toliopoulos, Anastasios Gounaris, Cosmas Vamvalis and
Daniel Caljouw. Event-based predictive maintenance on top of sensor data in a real Industry 4.0 case
study
15h50-16h00 Thiago Andrade, Brais Cancela and João Gama. Mining Human Mobility Data to Discover Locations and Habits
16h00-16h15 Barbara Bobowska, Jakub Klikowski and Michal Wozniak. Imbalanced data stream classification using hybrid data preprocessing
16h15-16h30 Mehmet Dinç, Åžeyda Ertekin, Hadi Özkan, Can Meydanlı and M. Volkan Atalay. Forecasting of Product Quality Through Anomaly Detection
16h30-16h45 Cristian Axenie, Radu Tudoran, Stefano Bortoli, Mohamad Al Hajj Hassan and Goetz Brasche. SPICE: Streaming PCA fault Identification and Classification Engine in Predictive Maintenance
16h45-16h55 Ehsan Aminian, Rita P. Ribeiro and Joao Gama. A Study on Imbalanced Data Streams
Keynote Talk

Myra Spiliopoulou
Myra Spiliopoulou is Professor of Business Informatics in the Faculty of Computer Science, Otto-von-Guericke-University Magdeburg. She leads the research group KMD on Knowledge Management and Discovery.
Her research is on the development and enhancement of data mining methods for person-web and person-person-via-internet interaction, for document analysis and for knowledge management. The main emphasis is on the dynamic aspects of data mining, including the evolution of behavioural patterns, thematic trends and community structures.
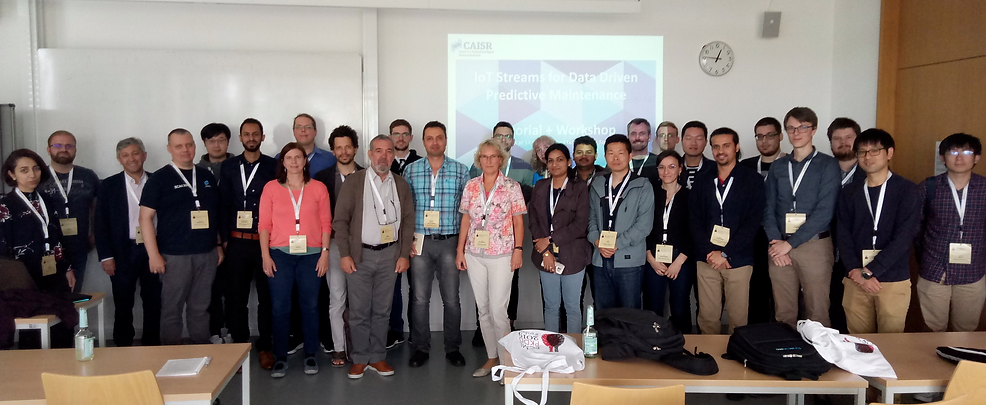